



个人介绍
卡内基梅隆大学硕士毕业,前滴滴高级算法工程师,现腾讯高级算法工程师。擅长图网络深度学习、时空序列问题、运筹优化等。现居北京。对人工智能的问题有很强烈的兴趣和解决的热忱,涉及到新领域的问题如区块链、智能医疗价格好商量。希望能在平台提供技术帮助的同时也能结交到不同领域的有志人才,用技术拓宽人脉。
工作经历
2020-08-26 -2021-09-03滴滴出行高级算法工程师
Developed and designed the graph structure and applied the graph traversal algorithm to generate geofences of carpool operation, which improved the match rate by 0.6 pp or 10% and decreased receipt payment ratio (rpr) by 0.3 pp • Designed a graph-rnn algorithm based on xgboost algorithm, which classified orders into good and bad categories, to predict the degree of each order as a recall condition to optimize the rpr by 0.3 pp • Explored multiple strategies of driver’s picking up allowance inclu
2019-07-14 -2020-05-24CMUResearch Assistant
Designed and developed OGCNN algorithm for material property prediction of Perovskite and Lanthanide alloys, achieved 94% improvement in MAEs of prediction for formation energy, 85% for bandgap, and 71% for fermi energy compared to MIT CGCNN algorithm in 2018 and submitted a paper on Physics Review Letter Journal
2019-01-01 -2019-06-20Lifeware LabsBioinformatics Machine Learning Researcher
• Developed Android app to plot and calculate Respiratory Rate from vibration sensor data in real time with Android Studio • Implemented peak-finder function for data stream for RR computing in Java with considerations of memory
教育经历
2017-08-23 - 2018-12-19Carnegie Mellon UniversityMaster of Science in Civil and Environmental Engin硕士
Selected Coursework: Machine Learning (PhD A), Convex Optimization, Stochastic Process, Advanced Graph Theory, Intermediate Statistics, Deep
技能
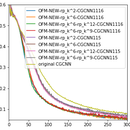
Designed and developed OGCNN algorithm for material property prediction of Perovskite and Lanthanide alloys, achieved 94% improvement in MAEs of prediction for formation energy, 85% for bandgap, and 71% for fermi energy compared to MIT CGCNN algorithm in 2018 and submitted a paper on Physics Review Letter Journal

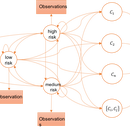
To effectively identify patients who may/may not be at risk for one or more postoperative complications in a dynamic, real-time setting, and generalize the detection to different types of surgeries, rather than an Early Warning Score [15] focusing on very specific complications, we propose to use pre-, intra- and post-surgery data to make interpretable risk predictions about who is likely to have complications, which complications may occur, and when in the postoperative setting.
